일 | 월 | 화 | 수 | 목 | 금 | 토 |
---|---|---|---|---|---|---|
1 | 2 | 3 | ||||
4 | 5 | 6 | 7 | 8 | 9 | 10 |
11 | 12 | 13 | 14 | 15 | 16 | 17 |
18 | 19 | 20 | 21 | 22 | 23 | 24 |
25 | 26 | 27 | 28 | 29 | 30 | 31 |
- 한화오션
- 프로그래머스
- TensorFlow
- Machine learning
- 정렬
- softmax
- 큐
- DFS
- deque
- join
- deep learning
- sort
- BOJ
- 백준
- Neural Network
- SQL
- 모두를 위한 머신러닝
- Programmers
- c++
- mysql
- 시간초과
- Queue
- 모두를 위한 딥러닝
- CSAP
- Linear Regression
- sung kim
- ML
- stl
- 알고리즘 고득점 kit
- PIR
- Today
- Total
목록mnist (2)
hello, world!
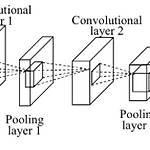
[Simple CNN] import numpy as np import tensorflow as tf import random mnist = tf.keras.datasets.mnist (x_train, y_train), (x_test, y_test) = mnist.load_data() x_test = x_test / 255 x_train = x_train / 255 x_train = x_train.reshape(x_train.shape[0], 28, 28, 1) x_test = x_test.reshape(x_test.shape[0], 28, 28, 1) # one hot encode y data y_train = tf.keras.utils.to_categorical(y_train, 10) y_test = ..
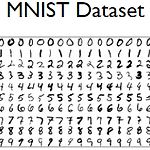
import tensorflow as tf learning_rate = 0.001 batch_size = 100 training_epochs = 15 nb_classes = 10 # download mnist dataset mnist = tf.keras.datasets.mnist (x_train, y_train), (x_test, y_test) = mnist.load_data() # normalizing data x_train, x_test = x_train / 255.0, x_test / 255.0 # change data shape print(x_train.shape) # (60000, 28, 28) x_train = x_train.reshape(x_train.shape[0], x_train.shap..