일 | 월 | 화 | 수 | 목 | 금 | 토 |
---|---|---|---|---|---|---|
1 | 2 | 3 | ||||
4 | 5 | 6 | 7 | 8 | 9 | 10 |
11 | 12 | 13 | 14 | 15 | 16 | 17 |
18 | 19 | 20 | 21 | 22 | 23 | 24 |
25 | 26 | 27 | 28 | 29 | 30 | 31 |
Tags
- Linear Regression
- Queue
- join
- Programmers
- deque
- 백준
- PIR
- softmax
- 프로그래머스
- DFS
- stl
- ML
- Machine learning
- sung kim
- 정렬
- TensorFlow
- SQL
- 큐
- Neural Network
- sort
- deep learning
- 시간초과
- BOJ
- 한화오션
- 알고리즘 고득점 kit
- mysql
- c++
- 모두를 위한 딥러닝
- 모두를 위한 머신러닝
- CSAP
Archives
- Today
- Total
목록deep nn (1)
hello, world!
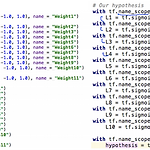
[Vanishing gradient] 위와 같이 9 layer 깊이 학습시켜보았다. poor results가 나왔다. 왜??? Backpropagation algorithm의 chain rule 때문에 그렇다. sigmoid function은 늘 0~1 사이의 값을 출력하기 때문에 layer가 깊어질수록 값이 작아진다. Vanishing gradient 발생! 경사도가 사라지는 현상이다. 첫 부분의 값이 최종 layer에 거의 영향을 미치지 못한다. 이 문제를 해결할 수 있을까? [Geoffrey Hinton] 1. We used wrong type of non-linearity 2. We initialized the weights in a stupid way 1. We used wrong type of..
AI/모두를 위한 ML (SungKim)
2021. 2. 24. 04:43